RAINIER is a statistical approach designed for fitting SEIR epidemic models to time series data, specifically applied to modeling COVID-19 transmission in Washington and Oregon. The approach leverages signal processing ideas to estimate susceptibilty, prevalence, transmission rates, and other hidden epidemiological quantities. RAINIER was used by the Washington Department of Health and the Oregon Health Authority to create weekly situation reports and to monitor the affects of various interventions in real time.
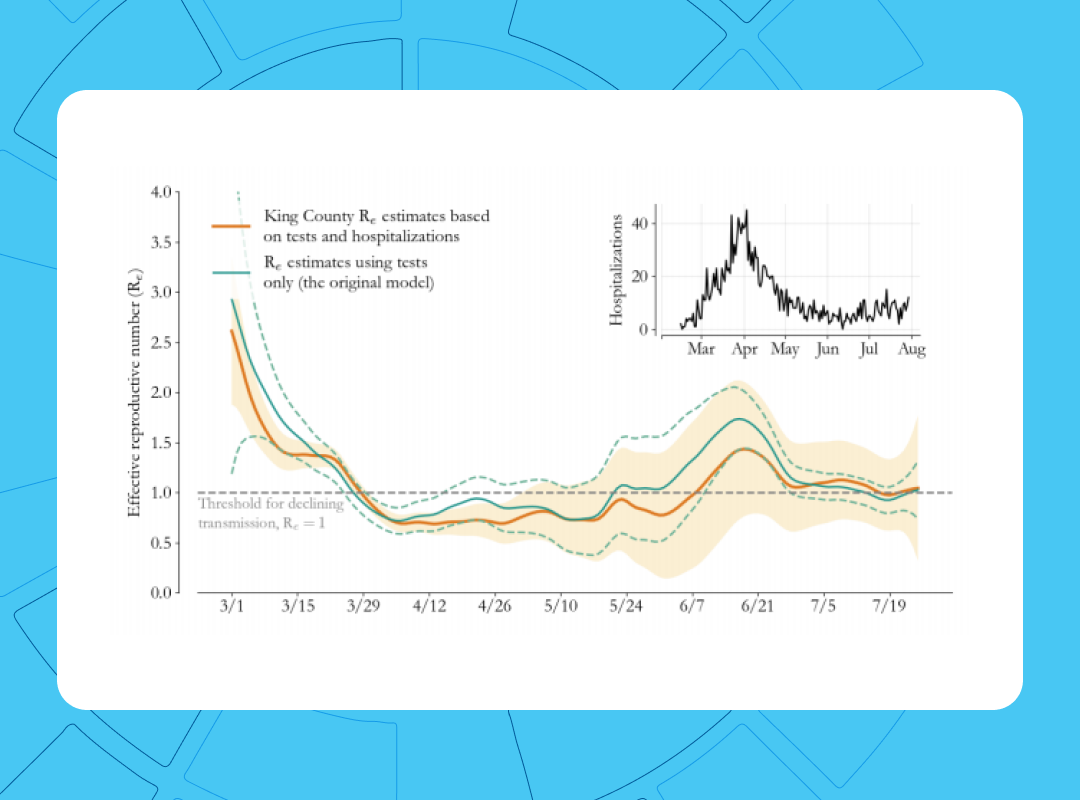
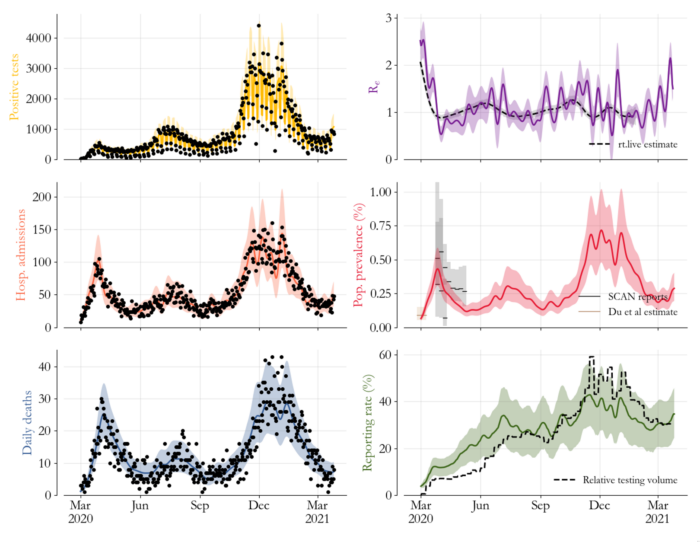
Features
Features of RAINIER include:
- Probabilistic approach for SEIR models: RAINIER is an inference method specifically designed to fit stochastic SEIR (Susceptible-Exposed-Infectious-Recovered) models, which are commonly used for simulating infectious disease dynamics.
- Alignment of model predictions with observed data: A key feature of RAINIER is its ability to adjust model predictions to closely match the actual observed case and mortality data, providing a more accurate understanding of pandemic dynamics.
- Application in real time: RAINIER is robust and fast, and was applied in real-time to COVID-19 data at county and state-levels successfully for the pandemic’s first two years.
- Application to COVID-19 scenario modeling: RAINIER has been particularly applied to modeling the transmission of COVID-19, with a focus on characterizing past interventions and exploring implications for future interventions.
Our approach
Our approach with RAINIER focuses on leveraging statistical rigor to create models of epidemic dynamics, particularly for COVID-19. By fitting SEIR models to both case and mortality data, we ensure that our models reflect real-world transmission patterns and outcomes. This method allows for the generation of modeling predictions, which can inform public health decisions and strategies.
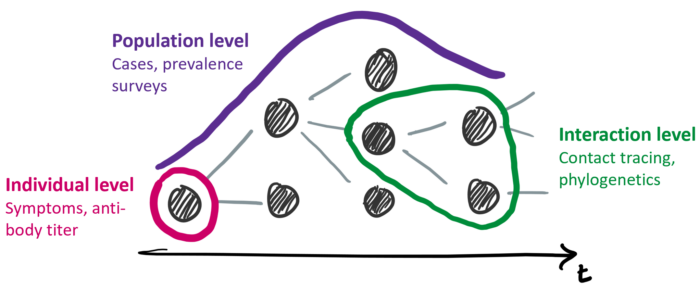