Mathematical models are invaluable for exploring the dynamics of infectious diseases, health delivery systems, intervention strategies, and other public health concerns. With technological advancements and the progression of AI, models are more accessible than ever before. However, it can be difficult to balance model selection, performance, and usability. IDM’s modeling methodology teams are working to improve the flexibility, capabilities, performance, and ease of use of models and workflows to better serve quantitative decision-making for our public health partners. By simplifying workflows and improving the quality of sharable models, we strive to facilitate the uptake of applied modeling in global health.
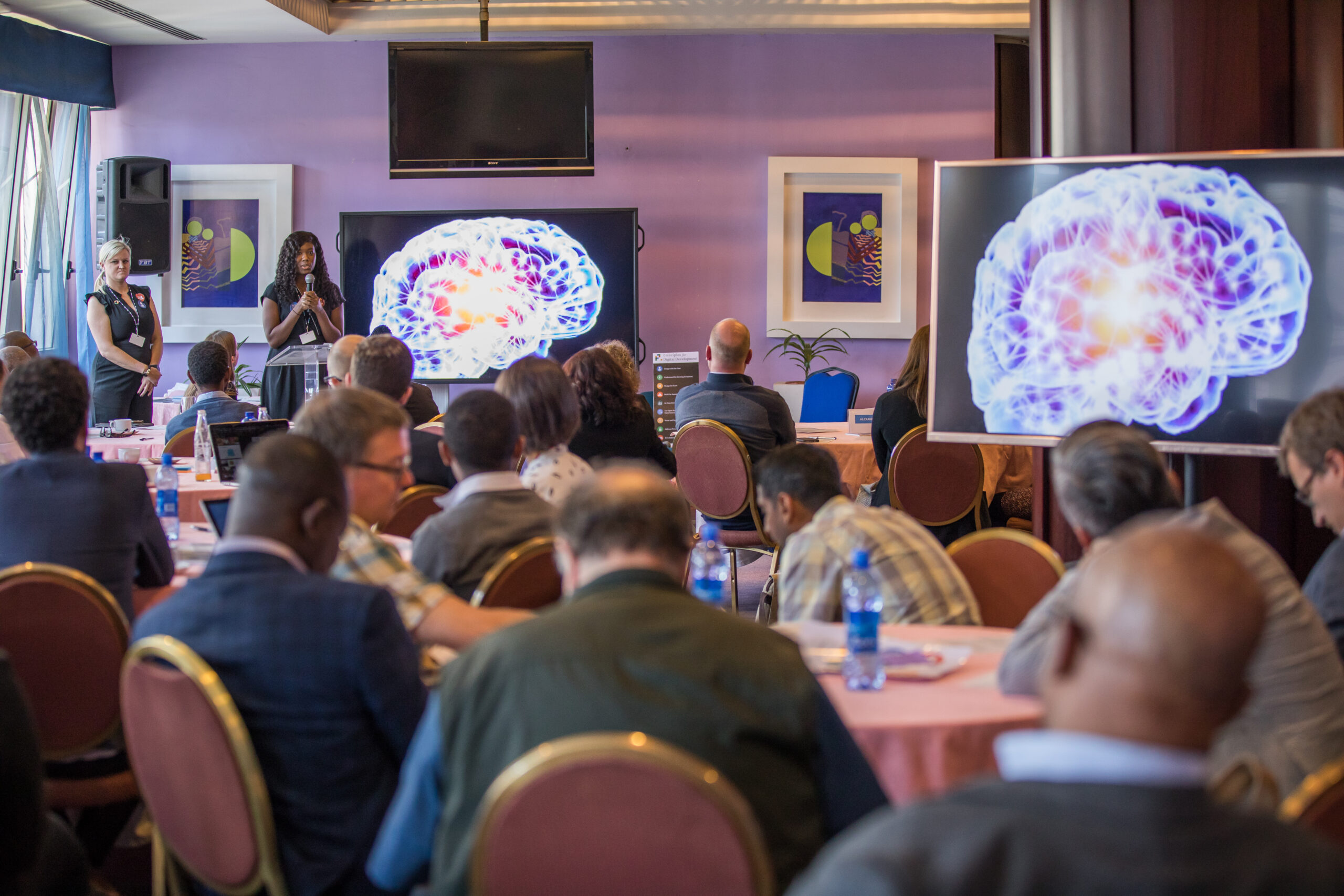
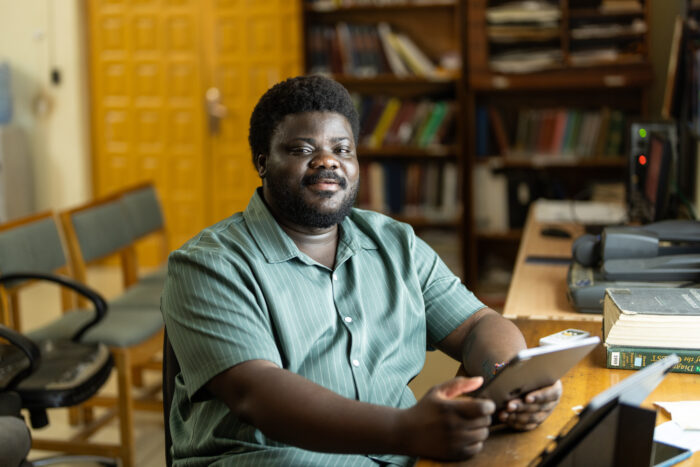
Our mission
The world is always changing with new tools, technology, and systems. We strive to improve the quality and quantity of applied modeling in global health. We do so by seeking out and developing novel model architectures, algorithms, and broader workflows to improve upon existing gaps in current standards and to increase the efficiency, capabilities, and uptake of modeling approaches.
Our approach
Modeling methodology encompasses a broad range of approaches. In general, we strive to increase the usability and quality of the tools we develop, both within IDM and for the external community. To increase accessibility, we conduct workshops on agent-based modeling techniques and disseminate documenation on modeling and our software.
Within dedicated functional teams, we adapt existing algorithms, methods, and model architecture to build effective new approaches to facilitate work across the breadth of IDM’s programmatic priorities. Example focal areas include:
- Designing user-centric approaches to model-configuration APIs and architectural patterns for specification of interactions between discrete modules (e.g. microbiome and nutrition on maternal outcomes, vaccine effectiveness, or enteric/respiratory disease progression)
- Refining algorithms and workflows for posterior estimation, scenario comparison, and model calibration — towards broader use in quantitatively evaluating structural model uncertainty, identifying sensitivity to key data gaps, and value of information of surveillance systems
- Exploring model design approaches for spatial surveillance and intervention-optimization use cases where the scale of the system is large and where the dynamics are dominated by the details of spatial topologies: heterogeneous risk, vaccine coverage, access to treatment, meta-population connectivity
- Advancing the state of phylogenetic summary statistics for inference and model calibration
- Relating advances in technology to opportunities to accelerate their application within global health, including advances in AI and LLMs to a diverse set of use cases as well as the uptake of machine learning related patterns in scientific Python and cloud compute to disease modeling contexts
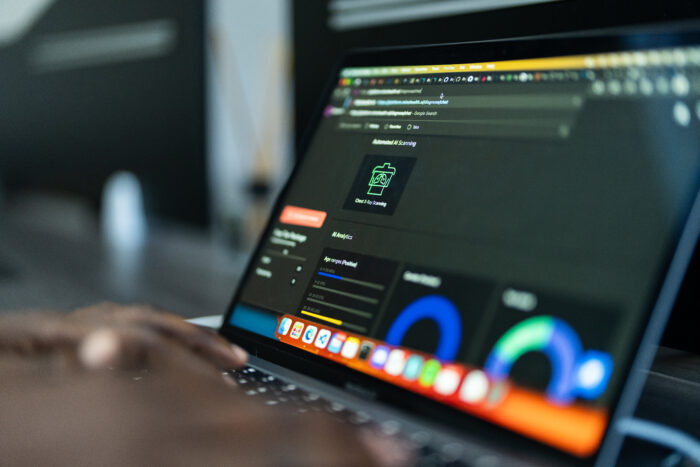
Publications
To learn more about IDM’s work on this topic, browse our publications!